Machine Learning
Contact
Head of lab
Lab Administration
Postal Address
Office Address
News
2 Sept 2024
We have presented the paper "Blind Zero-Shot Audio Restoration: A Variational Autoencoder Approach for Denoising and Inpainting", Boukun et al. (2024), at the Interspeech 2024 conference, Kos, Greece.
2 May 2024
We have presented the paper "Learning Sparse Codes with Entropy-Based ELBOs", Velychko et al. (2024), at the AISTATS conference in Valencia.
18 April 2024
Jörg Lücke gave the talk "On the Convergence and Transformation of the ELBO Objective to Entropy Sums" within the Math ML series at the MPI Mathematics in the Science / UCLA.
8 March 2024
Jan Warnken successfully defended his Master thesis. Congratulations!
4 March 2024
Jörg Lücke gave the talk "Are Large Models the Source of Intelligence?" at the MPI Biological Cybernics.
2 Feb 2024
Our paper Velychko et al. (pdf) has been accepted at AISTATS 2024.
23 Aug 2023
Our paper "Generic Unsupervised Optimization for a Latent Variable Model with Exponential Family Observables" (Mousavi et al.) has been accepted for publication by the Journal of Machine Learning Research.
22 Feb 2023
Joanna Luberadzka successfully defended her doctoral thesis. Congratulations!
20 Jan 2023
Our paper “The ELBO of Variational Autoencoders Converges to a Sum of Entropies” has been accepted for presentation at the AISTATS 2023 conference.
1 Dec 2022
The paper on sublinear variational clustering by Florian Hirschberger, Dennis Forster and Jörg Lücke has now been published by TPAMI.
4 Nov 2022
Jakob Drefs successfully defended his doctoral thesis "Evolutionary Variational Optimization for Probabilistic Unsupervised Learning". Congratulations!
21 Sept 2022
Jakob Drefs has presented his work at the ECML main conference.
14 June 2022
Our paper "Direct Evolutionary Optimization of Variational Autoencoders With Binary Latents" (Drefs et al.) has been accepted at ECML 2022.
23 March 2022
Yidi Ke has joined our lab. Welcome!
21 Jan 2022
Our paper "Evolutionary Variational Optimization of Generative Models" (Drefs et al.) has been accepted for publication by the Journal of Machine Learning Research (see here).
Machine Learning
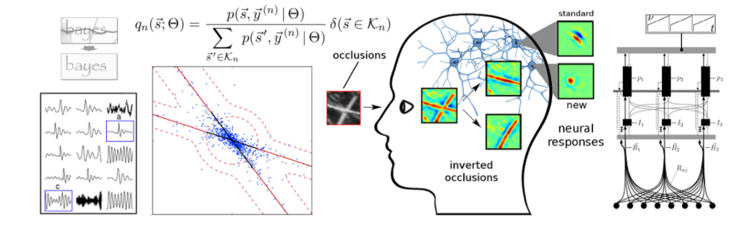
Our Research
Based on first theoretical principles, our group develops novel efficient learning algorithms for standard and novel data models. The resulting algorithms are applied to a range of different domains including acoustic data, visual data, medical data and data of general pattern recognition tasks. Alongside the theoretical and practical algorithm development, we investigate advanced Machine Learning methods as models for neural information processing; and, visa versa, use ideas and insights from the neurosciences to motivate novel research directions in Machine Learning.
We pursue and conduct projects on efficient generative models (including deep generative models) for large-scale unsupervised and semi-supervised learning, autonomous learning and data enhancement.
We are part of the cluster of excellence Hearing4all and the Department of Medical Physics and Acoustics at the School of Medicine and Health Sciences.
For any inquiries please contact Jörg Lücke.
Selected and Recent Publications
(The complete list can be found here.)
S.Salwig*, J. Drefs* and J. Lücke (2024).
Zero-shot denoising of microscopy images recorded at high-resolution limits.
PLOS Computational Biology 20(6): e1012192 (online access, bibtex)
*joint first authorship.
D. Velychko, S. Damm, Z. Dai, A. Fischer and J. Lücke (2024).
Learning Sparse Codes with Entropy-Based ELBOs.
Int. Conf. on Artificial Intelligence and Statistics (AISTATS), 2089-2097, 2024. (online access, bibtex)
H. Mousavi, J. Drefs, F. Hirschberger, J. Lücke (2023).
Generic Unsupervised Optimization for a Latent Variable Model with Exponential Family Observables.
Journal of Machine Learning Research 24(285):1−59. (online access, bibtex)
S. Damm*, D. Forster, D. Velychko, Z. Dai, A. Fischer and J. Lücke* (2023).
The ELBO of Variational Autoencoders Converges to a Sum of Entropies.
Int. Conf. on Artificial Intelligence and Statistics (AISTATS), 3931-3960, 2023. (online access, bibtex).
*joint main contributions
J. Drefs*, E. Guiraud*, F. Panagiotou, J. Lücke (2023).
Direct Evolutionary Optimization of Variational Autoencoders With Binary Latents.
European Conference on Machine Learning, 357-372. (pdf, bibtex).
*joint first authorship
F. Hirschberger*, D. Forster* and J. Lücke (2022).
A Variational EM Acceleration for Efficient Clustering at Very Large Scales.
IEEE Transactions on Pattern Analysis and Machine Intelligence 44(12):9787-9801 (online access, bibtex).
*joint first authorship.
J. Drefs, E. Guiraud and J. Lücke (2022).
Evolutionary Variational Optimization of Generative Models.
Journal of Machine Learning Research 23(21):1-51 (online access, bibtex).
J. Lücke and D. Forster (2019).
k-means as a variational EM approximation of Gaussian mixture models.
Pattern Recognition Letters 125:349-356 (online access, bibtex, arXiv)
A. S. Sheikh*, N. S. Harper*, J. Drefs, Y. Singer, Z. Dai, R.E. Turner and J. Lücke (2019).
STRFs in primary auditory cortex emerge from masking-based statistics of natural sounds.
PLOS Computational Biology 15(1): e1006595 (online access, bibtex)
*joint first authorship.
T. Monk, C. Savin and J. Lücke (2018).
Optimal neural inference of stimulus intensities.
Scientific Reports 8: 10038 (online access, bibtex)
A.-S. Sheikh and J. Lücke (2016).
Select-and-Sample for Spike-and-Slab Sparse Coding.
Advances in Neural Information Processing Systems (NIPS) 29: 3934-3942. (online access, bibtex)
Z. Dai and J. Lücke (2014).
Autonomous Document Cleaning – A Generative Approach to Reconstruct Strongly Corrupted Scanned Texts.
IEEE Transactions on Pattern Analysis and Machine Intelligence 36(10): 1950-1962. (online access, bibtex)
A.-S. Sheikh, J. A. Shelton, J. Lücke (2014).
A Truncated EM Approach for Spike-and-Slab Sparse Coding.
Journal of Machine Learning Research 15:2653-2687. (online access, bibtex)