PriMa - Privacy Matters
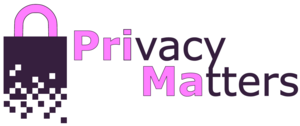
(this is a project together with the research team at the University of Twente in the Netherlands)
PriMa (Privacy Matters) is an Innovative Training Network (ITN) funded by the EU through the Horizon 2020 Framework. PriMa is a collaboration between 7 research locations and 7 industrial partner organisations with a focus on the analysis and mitigation of privacy risks in a rapidly digitising society. One factor contributing to the erosion of privacy is the growth in recognition technologies that not only facilitate the recognition of individuals but also the inference from biometric data of emotional state, gender, health, age, and even profession. Another factor is the fast advancement of artificial intelligence, allowing for extensive data mining, and aggregation, linkage and inference of personal information. Hence, there is a real possibility that acceptable privacy may become unattainable unless technological and societal steps are taken to allow citizens to regain control of their personal information.
In the context of the PriMa project, we are interested in the privacy protection of biometric templates. Established measures for privacy protection of stored biometric templates are (1) the classical template protection methods such as helper-data systems and (2) the more recent methods that perform biometric recognition under encryption. The first have the advantages that no key management is required, and computational complexity is low. The disadvantage is that the strength of the privacy protection is fully determined by the recognition performance of the biometric modality and for known modalities the secrecy rate does not exceed more than 20 bits, which is marginal from a cryptographic point of view. In the second approach the protection of privacy is decoupled from the biometric recognition performance and depends on the strength of the encryption, but this requires significant computational resources. In the past years, significant progress has been made in the field of homomorphic encryption using an optimal likelihood-ratio-based classifier. Protocols were developed for a 2-party scheme (a client containing the biometric sensor and a server containing the protected template) that are secure against a malicious attacker. Those schemes, however, still make the assumption that the registration of a subject is done in a trusted environment. Similar assumptions are made for the verification environment. Furthermore, the biometric accuracy of the homomorphic scheme completely depends on the accuracy of the underlying biometric feature vectors. Newly developed Deep Learning methods may be used to generate accurate feature vectors but may lead to privacy issues when trained models are published.
Our scientific goal therefore is to develop methods and minimal requirements for trusted enrolment (and possibly verification) in untrusted environments. Furthermore it will be considered if and how the use of Deep Learning techniques for generating biometric registration and verification feature vectors lead to privacy issues and how those can be prevented.