Topics for Theses
Contact
Prof. Dr. Claus Möbus
Room: A02 2-226
claus.moebus@uol.de
-------------------------------------------
Secretary
Manuela Wüstefeld
Room: A02 2-228
Tel: +49 441 / 798-4520
manuela.wuestefeld@uol.de
-------------------------------------------
Topics for Theses
Topics for Bachelor and Master Theses
Bachelor or Master theses in probabilistic modelling, machine learning or applied artificial intelligence are led by me and other researchers.
The work begins and ends with a lecture. In the initial presentation you introduce the topic and the milestone plan. This is done in my research seminar "Probabilistic Modeling" (inf 533 and/or inf534). You should register via Stud.IP for this seminar and also qualify for a certificate of successful participation. This can be achieved by the (successful) presentation and the written milestone description.
In the final presentation you summarize the results of the work (if necessary with a demonstrator). This takes place in the corresponding seminar ("upper seminar", etc.) of the co-investigator of the dissertation. Depending on the special field of the thesis (e.g. probabilistic robotics, computational intelligence, machine learning, business intelligence) the following colleagues (Prof. Fränzle, Prof. Kramer, Prof. Sauer) are recommended as co-examiners.
Interested students should consult this schedule ("Leitfaden") and are invited to contact me via email:
Prof. Dr. Claus Möbus claus.moebus@uol.de
Bayesian Portfolio Optimisation through Diversification of Risks
At the latest when prices collapse, some securities owners would wish they had done something to spread and minimise risk. According to Martin Weber (Professor at the University of Mannheim), a layman cannot perform better than the market, but he/she can do something for risk management in the portfolio. The classical non-Bayesian procedure was described theoretically by Nobel Prize winner Markowitz in 1952 in the article Portfolio Selection. Markowitz was awarded the Nobel Prize for this in 1990. In his book, Weber gives practical advice in chapter 6 of his book 'Genial einfach investieren; Mehr müssen Sie nicht wissen - das aber unbedingt !'. Somewhat more mathematical - but still easy to read - is the treatment of the topic of portfolio optimisation in Section 4.2 Diversification of Risks in the book by Cottlin & Döhler, Risikoanalyse, 2013 2/e. The algorithmic solution for the 2-ETF-case in the functional probabilistic language WebPPL can be found here.
In a previous BSc thesis the problem was solved up to a portfolio of 3 assets in the form of ETFs (see below news on this website). There are now two new challenges.
(1) In another BSc-thesis the optimization problem with more than 3 ETFs should be solved, the algorithm should be first implemented in the Julia programming language and in an interactive Pluto.jl-notebook and then evaluated for usability with financially affine laymen.
(2) In a MSc thesis students study how the classical approach of Markowitz can be extended the Bayesian way. This means that first priors over the portfolio components (here: ETFs) have to be assed from the preferences of financial investors. Then the to be developed algorithm computes the posterior distribution of the ETF shares in the portfolio. After that the user acceptance have to be assessed by behaviour measures.
A literature search is expected in the first part of the thesis. The book of RACHEV et al (2008) is a good starting point. In the second part realization possibilities are to be explored in the probabilistic programming language TURING.jl. In the last third a small demonstrator is to be built in an interactive Pluto.jl/Julia-notebook.
Challenges: There are two sets of challenges. The first are the normal challenges which arise when developing and implementing software for a stochastic optimization and decision procedure in a fixed amount of time. The second set of challenges is real scientific. It is grounded in cognitive science and user acceptance research. It has to be studied how the priors have to be obtained from financial investors and how satisfied they are with the posterios. Furthermore, the mechanism of the implementation has to be explained to the naive users so that they have maximal trust in the procedure so that they are willing to invest money in ETFs. We call a user naive when s/he has no knowledge about the inner workings of the algorithm or recommendation system.
Preknowledge: The candidate should have successfully studied WI, have a fundamental knowledge of applied statistics (mean, standard deviation, variance, correlation, regression, etc.), basic knowledge of stochastics (densities, distributions, likelihood functions, Monte-Carlo estimation,. etc), machine learning, understanding of chapter 6 of Weber's book, the prior BSc-thesis, and a working knowledge of Julia and Pluto.jl.
References
- COTTLIN & DÖHLER, Risikoanalyse: Modellierung, Beurteilung und Management von Risiken mit Praxisbeispielen, Heidelber: Springer, 2013 2/e.
- MÖBUS, C., Portfolio Optimization by Risk Diversification, 2018, here
- RACHEV, HSU, BAGASHEVA & FABOZZI, Bayesian Methods in Finance, Hoboken, New Jersey, Wiley, 2008
- TURING.jl,, Bayesian inference with probabilistic programming, 2022, https://turing.ml/stable/
- WEBER, M., Genial einfach investieren, Mannheim, 2015, [ebook](https://www.arero.de/fileadmin/user_upload/07_downloads/genial_einfach_investieren_ebook.pdf)
Interested students are invited to contact me via email:
Prof. Dr. Claus Möbus claus.moebus@uol.de
Efficient Algorithm for the Generation of the \(\textit{smallest}\) Sigma-Algebra \(\mathcal{A_E} = \sigma(\mathcal{E})\) conditional on a (known) Set System \(\mathcal{E}\)
"A σ-algebra ... is a set system in measure theory, i.e. a set of sets. A σ-algebra is characterized by its closedness with respect to certain set-theoretic operations. σ-algebras play a central role in modern stochastics and integration theory, since they appear there as definition domains for measures and contain all sets to which one assigns an abstract volume or probability, respectively" (Wikipedia, 2021/03/07)
Every primer on stochastics (Behrends, 2013, p.11; Hable, 2015, p.9; Halpern, 2017, p.14ff; Hübner, 2009, p.17) contains its definition and (e.g. Hable, 2015, p.10) present some trivial examples with countable sets (e.g. \(\Omega = \{1, 2, 3, 4\} \text{ or } \{a, b, c, d, e\}\)) or some very abstract and sometimes counterintuitive examples.
In empirical applications one is interested primarily in a special set \( \mathcal{E} \) of events which should be measured by probabilities. This set is in most cases not a full-fledged sigma-algebra. So to this set of interest \( \mathcal{E} \) more sets have to be added to "fill the gap" and to embed \( \mathcal{E} \) into a sigma-algebra. When this has been accomplished we say that "\(\sigma( \mathcal{E}) \) has been generated by set system \( \mathcal{E} \)". Some text books (e.g. Pollard, 2010, p.19f) provide small pedagogical examples with countable sets (e.g. \( \mathcal{E} =\{\{a, b, c\},\{c, d, e\}\}, \Omega=\{a, b, c, d, e\}) \).
From the view of mathematics the specification of \(\sigma\)- or \(\sigma(\mathcal{E})\)-algebras by their closedness is fully developed. This cannot be said about the constructive side. To our knowledge no stochastic textbook presents an efficient algorithm for the generation of the smallest \(\sigma(\mathcal{E})\). To this end we need intelligent strategies for sequencing the set-theoretic operations 'union' and 'complement'. Under 'strategy' we understand a set of rules of the type 'condition' \(\Rightarrow\)'action'.
Such an algorithm should be developed for the finite case in a BSc or MSc-thesis. Three examples demonstrate what outputs the algorithm should be generated from inputs \(\mathcal{E}\):
Example 1 (Hable, 2015, p.10)
input - the interesting set \(\mathcal{E}\) and \(\Omega\) - :
$$\mathcal{E} = \{ \{1\},\{2\}, \{1,2\},\{3,4\} \} \;\;\text{ and }\;\; \Omega = \{1, 2, 3, 4\}$$
output - \(\sigma\)-algebra (or \(\sigma\)-field) \(\sigma(\mathcal{E}) \subseteq \mathcal{P}(\Omega) \) with \( |\sigma(\mathcal{E})| = 8 < | \mathcal{P}(\Omega)|=2^4=16 \) - :
$$\sigma(\mathcal{E}) = \{\emptyset,\{1\},\{2\}, \{1,2\},\{3,4\},\{1,3,4\},\{2,3,4\},\{1,2,3,4\}\}.$$
where the set of atoms is: $$\{\{1\}, \{2\}, \{3, 4\} \}.$$
These 'atoms' are ideal because they are not further dividable, form a partition of \(\Omega\), and can be used as building blocks for other elements of \(\sigma(\mathcal{E})\).
Example 2 (Pollard, 2010, p.19f)
input - the interesting set \(\mathcal{E}\) and \(\Omega\) - :
$$\mathcal{E} = \{\{a, b, c\}, \{c, d, e\} \;\;\text{ and }\;\; \Omega = \{a, b, c, d, e\}$$
output - \(\sigma\)-algebra (or \(\sigma\)-field) \(\sigma(\mathcal{E}) \subseteq \mathcal{P}(\Omega) \) with \( |\sigma(\mathcal{E})| = 8 < | \mathcal{P}(\Omega)|=2^5=32 \) - :
$$\sigma(\mathcal{E}) =\{\emptyset, \{a, b\}, \{c\}, \{d, e\}, \{a, b, c \}, \{c, d, e\}, \{a, b, d, e\}, \{a, b, c, d, e\} \}.$$
where the set of atoms is: $$\{\{a, b\}, \{c\}, \{d, e\}\}.$$
These 'atoms' are ideal because they are not further dividable, form a partition of \(\Omega\), and can be used as building blocks for other elements of \(\sigma(\mathcal{E})\).
Example 3
input (Fig. 1) - the interesting set \(\mathcal{E}\) and \(\Omega\) - :
$$\mathcal{E} = \{E_1, E_2\} = \{\{a, b, c\}, \{a, b, e\}\} \;\;\text{ and }\;\; \Omega = \{a, b, c, d, e\}$$
output (Fig. 2) - \(\sigma\)-algebra (or \(\sigma\)-field) \(\sigma(\mathcal{E}) \subseteq \mathcal{P}(\Omega) \) with \( |\sigma(\mathcal{E})| = 2^4=16 < | \mathcal{P}(\Omega)| = 2^5=32 \) - :
$$\sigma(\mathcal{E}) =\{\emptyset, \{c\}, \{d\}, \{e\}, \{a, b\}, \{c, d\}, \{c, e\}, \{d, e\}, \{a, b, c\}, \{a, b, d\}, \{a, b, e\}, \{c, d, e\}, $$
$$\{a, b, c, d\}, \{a, b, c, e\}, \{a, b, d, e\}, \{a, b, c, d, e\}\}.$$
where the set of atoms is: $$\{\{a, b\}, \{c\}, \{d\}, \{e\}\}.$$
These 'atoms' are ideal because they are not further dividable, form a partition of \(\Omega\), and can be used as building blocks for other elements of \(\sigma(\mathcal{E})\).
An algorithmic solution sketch was provided in his book (Behrends, 2013, p.16) and in a personal communication (Behrends, 2021/03/04) "...this is an interesting question for which I know of no theoretical research. I think that the complexity grows strongly exponentially. More precisely it looks like this. Let \(\Omega\) have \(r\) elements, let \(k\) subsets \(E_1,...,E_k\) be given, and one is interested in the generated sigma algebra \(\Sigma\) of \(E_i\). To do this, one must know the atoms of \(\Sigma\), the minimal nontrivial elements. If there are \(n\) pieces, \(\Sigma\) has \(2^n\) elements.
And how do you find the atoms? The easiest way is by induction on \(k\). For \(k=1\) there are (at most) 2 atoms, and at the transition \(k \rightarrow k+1\) one has only to form the intersections with \(E_k\) and \(\Omega\setminus E_k\) of the atoms to \(k-1\). In short: There will be at most \(2^k\) atoms, i.e. \(\Sigma\) can have \(2^{2^k}\) elements
And this can happen in the worst case. For example, if \(\Omega=\{0,1\}^s\) and one chooses \(E_i\) as "i-th entry equals 1" for \(i=1,..,s\), the atoms are the one-element sets, so there are \(2^s\) atoms. Unfortunately, I cannot contribute further subtleties. For example, how elaborate is it to compute the intersection of two subsets of an r-elementary set? I guess \(2r\) steps, and thus we end up with an effort of \(2r \cdot 2^{2^k}\)." (personal email of E.Behrends, 2021/03/04)
In a BSc-thesis questions 1-2 have to be answered, the algorithm for generating \(\sigma(\epsilon)\) should be formulated in pseudocode, its complexity should be specified, and implemented in Julia and Pluto.jl-notebooks. The generated \(\sigma(\epsilon)\) should be made visible in graphics (similar to those shown below) embedded in a Pluto.jl-notebook. The implementation should exploit parallel features of Julia and the accumulate-filter-generate pattern.
In a MSc-thesis in addition to the achievements of a BSc-thesis the algorithmic idea should if possible transferred to the transfinite domain. A near algorithmic solution sketch is provided in Behrends (2013, p.16, p.29f).
Computer science (informatics) students interested in this topic should apply with a proof of their competence in stochastics and machine learning.
References
- BEHRENDS, E., Elementare Stochastik - Ein Lernbuch, von Studierenden mitentwickelt - , Springer Spektrum, 2013
- HABLE, R., Einführung in die Stochastik - Ein Begleitbuch zur Vorlesung - , Springer Spektrum, 2015
- HALPERN, J.Y., Reasoning About Uncertainty, 2/e, MIT Press 2017
- HÜBNER, G., Stochastik - Eine anwendungsorientierte Einführung für Informatiker, Ingenieure und Mathematiker, 5.Auflage, Vieweg-teubner, 2009
- POLLARD, D., A User's Guide to Measure Theoretic Probability, Cambridge University Press, 2010
Computer science (informatics) students interested should consult this schedule ("Leitfaden") and are invited to contact me via email:
Prof. Dr. Claus Möbus claus.moebus@uol.de
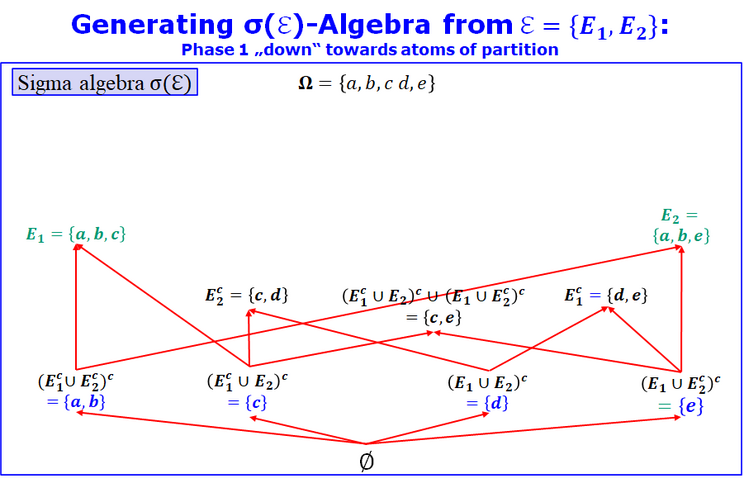
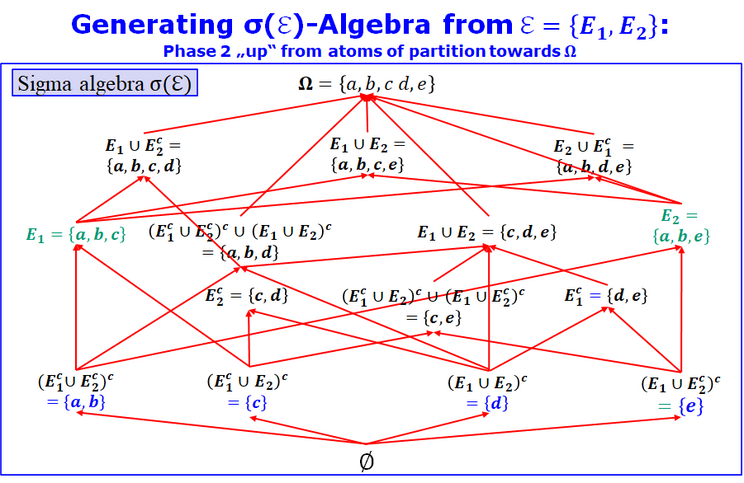
Paradigmatic Problems for the Probabilistic Programming Languages TURING.jl and WebPPL
WebPPL and Turing.jl are relatively new Turing-complete universal probabilistic programming languages (PPLs). WebPPL is embedded in the functional part of JavaScript (JS). Turing.jl is embedded in Julia. Both WebPPL and Turing.jl are open source and experimental.
PPLs are used for building generative probabilistic models (GPMs). These models represent causal background knowledge which is characteristic for experts. In contrast, deep learning models only represent shallow knowledge which is useful for pattern matching and computer vision. In that respect the latter have become rather successful. This paper describes with many examples the fundamental difference between DL and GPMs (Lake, et al., 2016).
The thesis should survey models programmed in WebPPL and Turing.jl according to a metric measure (e.g. code length). This set is called the paradigmatic example set WebPPL-problems \(\cup\) Turing.jl-problems. This set should be partitioned in the joint set WebPPL-problems \(\cap\) Turing.jl-problems and the two difference sets Turing.jl-probs \ WebPPL-probs and WebPPL-probs \ Turing.jl-probs. The last two sets are of special interest. Are there these sets by chance or are there fundamental difficulties in formulating a problem solution in one of the two languages?
Let's take the example of "penalty kicks" from the football world. There are two agents in the penalty shootout. The goalkeeper and the shooter. Each agent has certain preferences for certain shots and certain defensive measures: e.g. left upper corner, etc.. At the same time, each agent has guesses about the opponent's preferences. We know from WebPPL that the language means are sufficient to model such situations. But what about Turing.jl ?
Such a question should be clarified in the work.
Interested students are invited to contact me via email:
Prof. Dr. Claus Möbus claus.moebus@uol.de
Probabilistic Modeling with Model Fragments, Patterns, or Templates
WebPPL is a web-based probabilistic programming language (PPL) embedded in JavaScript. PPLs are used for implementing probabilistic models in domains with uncertain knowledge (cognition, medicine, traffic, finance, etc). Dependent on the situation-specific problem questions in the form of unknown (conditional) probabilities are formalized. Models and programs generate answers by numerical inference processes.
The interactive tutorial "Probabilistic Models of Cognition" provides a variety of WebPPL-models. There is nearly always a fixed sequence modelling steps: 1) modelling the causal process of interest (root causes, expositions -> syndroms -> symptoms), 2) observation of evidence (data), 3) (diagnostic) inference (most often) contrary to the causal direction (symptoms -> syndroms -> expositions).
Challenge: The research question is, whether the modelling process can be improved substantially by a library of model fragments, patterns, or templates.
Prerequisites: The topic is suited for a master thesis. Preknowledge can be acquired by successful participation in the seminar "Probabilististic Modeling I & II" (Inf533, Inf534) and studying the above mentioned tutorial.
Contact: Prof. Dr. Claus Möbus